Financial reports used to mean waiting. Waiting for data entry, waiting for reconciliation, waiting to spot problems until they became expensive mistakes. AI eliminated that entire cycle. Now insights flow in real-time, errors get caught immediately, and stakeholders get answers when they ask—not three weeks later during month-end close.
The impact touches everything. Companies make decisions faster because they can. Investors analyze opportunities the moment they appear. And organizations stuck in old patterns? They struggle to keep up with a market that doesn't wait anymore.
Key Takeaways
- AI ignites efficiency and accuracy in your financial reporting.
- Companies need to evolve with AI if they expect to stay competitive.
- Understanding AI's role in finance is critical for strategy and decision-making.
Evolution of Financial Reporting
Technology always promised to improve reporting. AI actually delivered.
Historical Context and the Advent of AI
Paper dominated finance for generations. Manual entries, endless verification, constant errors that took days to find and weeks to fix. The whole system ran on human effort and human limitations. Nobody loved it. Everyone accepted it.
Spreadsheets brought the first real change. Finally teams could automate calculations, reduce basic errors, build something resembling efficiency. It felt revolutionary at the time. Now it looks like a baby step.
AI redrew the map entirely. Massive datasets process in seconds. Complex patterns surface automatically. Tasks that used to consume entire departments now happen in the background while teams focus on what the numbers mean instead of just collecting them.
The Role of AI in Modern Financial Reporting
Believe it or not, most companies have already integrated AI into their financial processes in some way. Not necessarily because they wanted to. Because they had to. Modern business generates too much data for traditional methods. The systems handle everything from basic entry to complex reconciliation while spotting problems humans might miss entirely.
Not everyone embraced the change. Security concerns keep executives cautious. Training takes time and money. Valid issues, all of them. But while some organizations hesitate, others pull ahead. Modern finance runs on AI. Everything else just falls behind.
AI Technologies in Financial Reporting
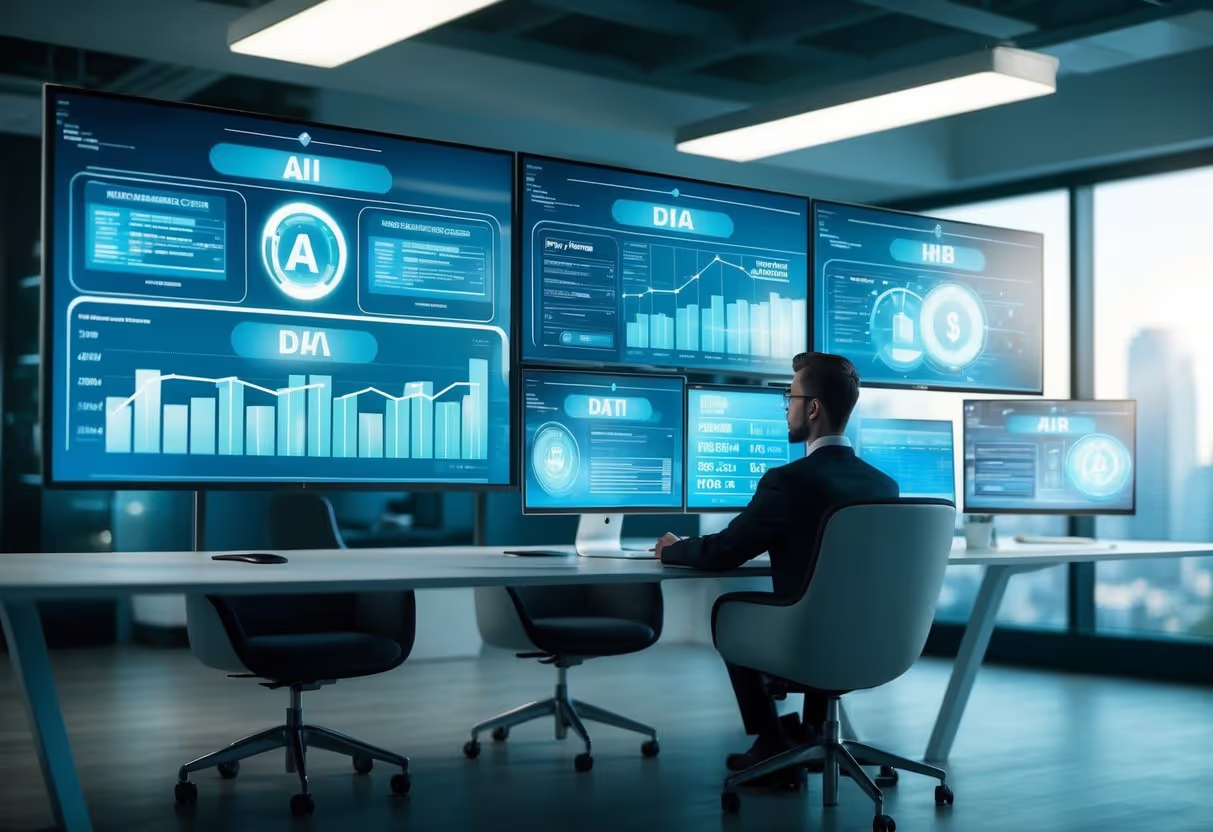
Old tools track data. New ones understand it. The difference matters more than most organizations realize.
Machine Learning Algorithms
Machine learning drives everything in modern financial reporting. It processes data faster than humans ever could, finds patterns we might miss, predicts outcomes based on countless variables. The systems learn from every analysis, getting sharper over time.
Natural Language Processing and Generation
Numbers tell half the story. Text holds the rest. NLP changed how we handle all that unstructured data floating around financial systems—earnings reports, market updates, internal documentation. The technology reads through it all, extracts what matters, connects dots humans might never link.
The systems don't just read anymore—they write. Preliminary analyses appear automatically. Draft reports generate themselves. Professionals spend time refining insights instead of formatting documents. The whole process moves faster, surfaces better insights, keeps teams focused on analysis instead of administration.
Predictive Analytics and Data Analysis
Past performance can help gauge future results. In theory. Predictive analytics makes those predictions actually mean something. The systems analyze historical trends, factor in current conditions, project likely outcomes based on real data instead of gut feelings.
Organizations use this for everything now—assessing market conditions, tracking customer behavior, evaluating investment risks. Resource allocation happens based on data, not departmental politics. And the whole system gets more accurate with every cycle.
Anomaly Detection Systems
Traditional monitoring couldn't keep up with transaction volume. Problems stayed buried until month-end reviews or external audits caught them. Modern detection systems monitor everything in real time instead. Unusual patterns trigger alerts immediately. Small issues get addressed before they grow into expensive problems.
It made reporting more reliable too. Teams investigate anomalies right away. Compliance issues surface when they're still fixable. The whole system runs smoother because nothing hides in the background anymore.
Benefits of AI in Financial Reporting
The practical impacts matter most. Here's what changed in actual practice.
Enhancing Accuracy and Reducing Human Errors
Data validation used to eat up half our time. Manual checks, endless reconciliation, constant mistakes that took days to find. Modern systems connect directly to data sources, run checks automatically, keep everything consistent across all your systems. The basic errors that used to plague every reporting cycle just don't happen anymore.
Machine learning spots the problems we always missed in manual reviews. Outliers pop up immediately. Patterns emerge across millions of transactions. Finance teams work with clean data instead of wondering what they missed.
Increasing Efficiency and Productivity
Report preparation used to paralyze entire departments. ERP systems that didn't talk to each other. Spreadsheets that never matched. Modern platforms eliminate that entire headache. They handle all the routine work—data collection, validation, preliminary analysis.
The reporting cycle looks completely different now. Data flows automatically between systems. Teams spot issues and adjust course immediately instead of discovering problems during month-end close. Companies that upgraded their systems pull further ahead every quarter while the rest drown in manual processes.
Improving Risk Management and Compliance
Risk management used to mean damage control. Something went wrong, then teams scrambled to fix it. Modern systems catch problems upstream before they cause real damage. Compliance stays on track automatically because nothing slips through the cracks anymore.
The time saved transformed how teams operate. People who spent their days monitoring basic compliance now focus on actual business value. Issues get fixed while they're still manageable. The whole system runs smoother because prevention replaced reaction.
Gaining Strategic Insights and Forecasting
Trend analysis meant best guesses based on limited samples. Modern analytics process every transaction your company ever made. The systems find connections humans would never spot, predict outcomes based on complete data instead of hunches.
Planning evolved completely. Teams model different scenarios using real data from across the business. The forecasts mean something because they're built on actual analysis instead of assumptions. Better data drives better decisions because everyone finally sees the whole picture.
Application of AI in Different Reporting Functions
The theoretical benefits sound impressive. But what happens in actual practice? The applications tell the real story.
Automation of Data Collection
Data collection used to mean manual input and constant errors. AI extracts information directly from invoices, transaction records, bank statements - any source an organization uses. The systems standardize everything automatically, improve accuracy dramatically, reduce labor costs across the board.
Traditional data entry tied up entire teams. Now those same professionals focus on analyzing the data instead of inputting it. Reports generate faster. Quality improves consistently. The systems learn from every interaction, getting more accurate over time.
Real-Time Monitoring and Updates
End-of-month reporting created too many surprises. Someone would spot a problem, but the damage was already done. Modern systems track everything as it happens. Financial data flows between departments automatically. Issues surface while you can still fix them.
Cash flow management became proactive instead of reactive. Teams see problems developing and adjust course immediately. The monthly review cycle that everyone hated just disappeared because continuous monitoring works better.
Fraud Detection and Anomaly Identification
Traditional fraud detection always worked backward. By the time you spotted something wrong, the money was gone. Modern systems track patterns across every transaction. They spot suspicious activity immediately and adapt to new fraud tactics automatically. Prevention finally replaced investigation.
Financial Statement Analysis and Preparation
Statement preparation used to consume entire accounting departments. Pulling data from different systems. Validating everything manually. Running endless reviews. Modern platforms connect to every data source automatically. They handle validation, generate accurate reports, surface trends that matter—all while you grab coffee.
The focus shifted completely. Teams spend time analyzing what the numbers mean instead of just collecting them. Cash flows, income statements, balance sheets - everything processes automatically. The machines handle data collection while humans handle insights.
Challenges and Considerations
New technology always brings headaches. But AI's challenges surprised everyone. The tools work fine—it's the implementation that gets messy. Getting systems talking to each other, training teams on new processes, maintaining data quality - that's where the real work happens.
Balancing Human Judgment and Machine Intelligence
AI calculates faster than humans ever could. Processes more data. Spots more patterns. But it misses context completely. Miss the human element, and you miss everything that matters.
Some firms went too far with automation. Learned hard lessons. Because AI handles the math brilliantly—but judgment calls need human insight. Smart teams learned to leverage both. Let machines do what machines do best. Let humans focus on the decisions that need human understanding.
Addressing Data Privacy and Ethical Concerns
Breaches cost millions. Reputation damage costs more. Every organization running AI in finance faces the same reality—protect the data or pay the price.
Bias creeps into algorithms. Always has. Regular audits catch most issues, but transparency matters more. Because when AI makes financial decisions, everyone needs to understand why. No black boxes. No hidden processes. Just clear paths from data to decisions.
Managing Operational and Regulatory Risks
Systems fail. Updates break things. Training gets delayed. That's reality. But financial AI can't afford downtime—the cost runs too high.
Regulations change constantly. Each update brings new requirements, new standards, new ways to fall behind. Finance teams learned to build flexibility into everything. Because rigid systems break under regulatory pressure. Adaptive ones survive.
Future of AI in Financial Reporting
Nobody predicted how fast this would change. Or how completely. What's coming looks even bigger.
Trends and Developments to Watch
Large language models shifted everything again. Reports that took days now take minutes. Analysis that needed teams now happens automatically. And automation? It's touching parts of finance nobody thought machines could handle.
The gap keeps growing. Modern systems pull further ahead every quarter. Traditional approaches can't keep up—they're fighting a battle that ended years ago.
Evolving Regulatory Landscape and Governance Policies
Privacy rules changed the game entirely. Every quarter brings new requirements. New restrictions. New ways to fail compliance. Standing still isn't an option anymore.
Governance got messier too. Financial AI needs guardrails—clear policies about data usage, algorithmic decisions, automated processes. The oversight burden grows heavier every year. But the alternative costs more.
Preparing for the Next Wave of AI Advancements
Training never ends anymore. New tools, new capabilities, new ways to leverage AI—teams either keep learning or fall behind. No middle ground exists.
Integration doesn’t happen overnight. Getting AI tools to work with legacy systems takes time, money, patience. But the ones who figure it out first gain advantages that last.
Still, integration challenges persist. Organizations work to merge AI solutions with existing systems, create seamless workflows, build processes that actually work in practice. Those that prepare now position themselves to benefit from whatever comes next.
Want to simplify your reporting process? Streamline your audit preparation? InScope helps you leverage automation and AI to eliminate manual work and reduce errors, keeping both regulators and stakeholders happy. When you're ready to spend less time wrestling with spreadsheets and more time analyzing results, check out what InScope can do—request a demo today.
FAQs
1. How is artificial intelligence revolutionizing financial statement analysis?
Financial statement analysis used to mean long nights with spreadsheets, hoping you caught everything important. Now AI processes the entire financial history of your company in minutes. It spots trends and problems humans always missed just because we couldn't process that much data. Every transaction gets analyzed instead of relying on samples.
2. What are the implications of machine learning in the accuracy of financial reporting?
Basic errors used to slip through all the time. Copy something wrong, transpose a number, miss a decimal point—the whole report could be off. Machine learning eliminated those headaches. The systems handle all the validation automatically and get smarter with every transaction they process. No more fixing simple mistakes.
3. What are the capabilities of AI-powered tools for generating financial reports?
Remember when report generation meant copying data between systems for days? Modern tools connect to everything—your ERP, accounting software, sales platforms. They pull what they need automatically, validate it, format it correctly. No more manual checks. No more data entry. Just clean reports ready for analysis.
4. Can artificial intelligence systems effectively summarize complex annual reports?
Have you ever tried reading through five years of annual reports to spot trends? AI handles that in seconds. It pulls out the key information, connects related points across different sections, highlights what actually matters. Analysts focus on understanding the business instead of just finding the right numbers.
5. How does the integration of AI in financial reporting impact compliance and regulatory standards?
The old approach to compliance never worked well enough. Check things periodically, hope you catch problems before the auditors do. Modern systems monitor everything continuously. They maintain audit trails automatically. Catch issues early. Nothing has time to become a serious problem because the oversight never stops.