AI is already transforming audits, from the ground up. Firms use it to analyze massive amounts of data in ways that weren't possible before. What used to take weeks now happens in hours. And the improvements go deeper than just saving time—AI fundamentally changes how firms approach governance, risk management, compliance.
These tools give auditors capabilities that seemed impossible just a few years ago. They spot patterns earlier. They manage risks better. They adapt faster to new requirements. For firms that haven't embraced these changes yet, the gap widens every day. Modern auditing simply demands these capabilities now.
Key Takeaways
- Combining AI with advanced data analysis can significantly improve audit efficiency and accuracy.
- Technology constantly creates new ways to help auditors manage risks and ensure compliance.
- AI already has a central role in audit practices, and will only get more essential over time.
Fundamentals of AI in Auditing
To put it mildly, AI is changing rapidly in audit. Some AI-driven capabilities become outdated almost instantly. Others stay essential year after year. Such rapid change means it’s important for businesses to understand what AI in auditing is really about—a distinction that makes the difference between chasing features and getting results.
Defining AI and Its Role in Auditing
AI handles tasks that humans used to spend hours on. It processes thousands of transactions in minutes, spots patterns buried in financial data, flags anomalies that need attention. Manual reviews always missed things—they had to. The sheer volume made it impossible to catch everything. Now, auditors focus on analyzing problems instead of hunting for them. When potential risks surface, they surface early.
Types of AI Technologies Relevant to Auditing
Machine learning sits at the heart of modern auditing. The algorithms learn from data and improve their performance over time. Natural language processing evaluates textual data—contracts and audit reports that used to require manual review. Robotic process automation streamlines repetitive tasks, giving auditors more time for complex problem-solving.
Understanding the Audit Lifecycle
Audits still move through the same stages they always have. But the work inside those stages looks completely different. AI plans better because it sees the whole picture at once. Fieldwork covers more ground because machines handle the routine tasks. Reports come together faster, show more detail. Follow-up catches problems earlier.
Role of Machine Learning and Data Analytics
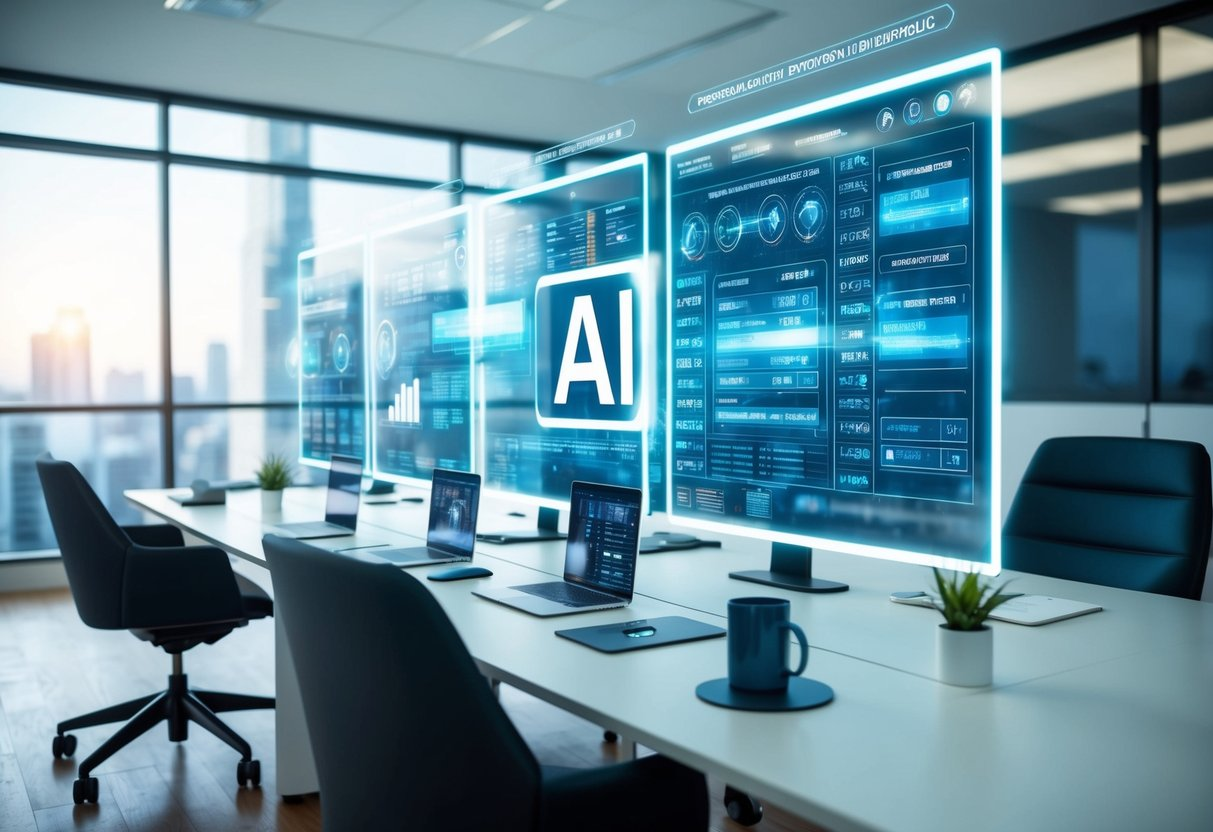
Most auditors already use machine learning without thinking about it. The algorithms run in the background, finding patterns, flagging outliers. Analytics tools show trends that used to take weeks to spot.
Application of ML in Audit Processes
Machine learning transforms how auditors handle routine work. Take journal entry testing—ML algorithms spot unusual transactions automatically in massive datasets. This automation shifts auditors' attention where it belongs—to high-risk areas that need professional judgment. The models keep processing more data, adapting to new scenarios, improving their effectiveness. Every audit gets faster, more reliable.
Leveraging Data Analytics for Insightful Auditing
Data analytics opens up new possibilities in financial record analysis. Visualization tools turn complex data into clear insights. Statistical methods uncover meaningful trends across time periods. Teams share findings instantly, collaborate better, make decisions based on solid data.
Traditional barriers disappear when everyone sees the same information. Trends emerge faster. Problems surface sooner. The entire process flows more smoothly when analytics drive the insights.
Predictive Models in Risk Assessment
Historical data powers modern risk assessment. Predictive models analyze past patterns to forecast potential issues, helping firms direct resources exactly where they're needed. But models need context—market conditions, industry trends, organizational changes all matter. Surface-level analysis misses crucial nuances.
The technology keeps evolving, making predictions sharper and more reliable. Yet many firms barely scratch the surface of what's possible. They run basic trend analysis and stop there. Real predictive power comes from combining historical patterns with deep business understanding.
Enhancing Audit Quality with NLP and RPA
Natural language processing and robotic process automation change the fundamentals of audit quality. These technologies handle previously manual tasks with unprecedented speed and accuracy. Every aspect of documentation, analysis, and reporting transforms.
NLP for Advanced Data Processing
Text data used to create bottlenecks. Contracts, emails, reports—mountains of unstructured information that needed human eyes. NLP changes that dynamic entirely. It evaluates documentation efficiently, spots patterns in vendor communications that might signal fraud, identifies key information buried in text. Auditors shift from searching through documents to focusing on strategic assessment and decision-making.
RPA in Streamlining Audit Processes
Robotic process automation eliminates the tedious parts of auditing. Data entry, reconciliation, reporting tasks—work that used to consume hours happens automatically. Real-time access to information changes how teams work. Human error in routine tasks disappears. Auditors focus on complex areas that actually need human judgment and expertise.
Automating Compliance and Reporting
NLP and RPA transform compliance monitoring and reporting together. NLP analyzes compliance documentation, ensuring all standards get met. RPA generates reports automatically from this analyzed data, reducing oversight risk dramatically. The entire process becomes simpler, less stressful.
Audit Planning and Execution
Planning meetings look different these days. Instead of guessing where problems might hide, teams see them highlighted automatically. Resource decisions get easier when data shows exactly where attention needs to go.
Integrating AI into Audit Planning
Computers spot potential issues faster than humans ever could. They process years of transactions in minutes, flag patterns that need attention. Audit teams spend less time searching for problems, more time solving them.
AI-Driven Journal Entry and Population Testing
Nobody tests samples anymore—they don't have to. AI reviews every transaction, spots irregularities instantly. The system flags unusual patterns for human review. Nothing gets missed because of sample size limitations.
Digital Transformation in Audit Execution
Most firms work differently now. AI tools process data as it comes in, letting teams adjust their approach on the fly. Productivity improves naturally. Compliance gets easier to maintain. Thanks to digital transformation, firms adapt faster to industry changes without sacrificing quality.
AI Systems and Internal Control
Internal controls used to depend heavily on human oversight. Not anymore. Automated systems catch issues that manual monitoring might miss. The whole process runs smoother, faster, more reliably.
Strengthening Internal Audit with AI Solutions
Anomalies stand out immediately in AI-processed data. Transactions that need attention get flagged automatically. Control weaknesses surface before they cause problems. Internal audit teams see the whole picture clearer, respond faster when issues emerge.
Teams communicate findings differently now. Stakeholders see the same data at the same time. Audit teams adjust faster when issues surface. The whole process just works better.
Controls for AI Risk Management
AI systems need their own controls. Every organization needs clear frameworks defining roles and responsibilities. Data integrity matters most—AI models only work as well as the information feeding them.
Model validation keeps algorithms on track and aligned with goals. Ethics guidelines prevent common problems like bias in automated decisions. Organizations that handle these aspects well get more value from their AI investments.
Audit Committee Reporting Enhancements
Committee reporting looks different with AI analytics. Customizable reports highlight risk areas the moment they appear. Dashboards turn complex data into something committees can actually use.
Monitoring happens continuously now, not just during periodic reviews. Committees spot emerging risks and control weaknesses earlier. Meetings focus on actual issues instead of reviewing old reports.
Governance, Risk, and Compliance
AI changes how organizations handle GRC functions. Analysis that took weeks now happens immediately. Patterns emerge faster. Problems surface sooner. Everything about risk management and compliance moves at a different speed.
Risk Assessments Powered by AI
Data collection happens automatically now. AI spots trends and potential threats across operations. Risk managers spend less time gathering information, more time deciding what to do about it.
Predictive analytics shows where problems might develop. Resources flow to high-risk areas naturally. Teams spend time on solutions instead of assessments.
Regulatory Compliance and AI in Audits
Keeping up with regulations takes constant attention. AI monitors changes continuously, flags potential compliance issues early. When discrepancies appear in large datasets, they surface automatically.
Audit processes stay streamlined. Error rates drop. Compliance reports show regulators exactly what they need to see. Everything stays documented, verified, ready for review.
Governance Supported by Intelligent Insights
Leadership sees performance metrics in real-time now. Strategy adjustments happen faster. AI spots patterns in governance practices that traditional reviews might miss.
The reporting process stays automatic. Information reaches decision makers when they need it. Organizations align their governance frameworks with actual operational needs instead of theoretical best practices.
Security, Transparency, and Accountability
Security matters more than ever with AI systems. Organizations handle more data, process it faster, share it more widely. That creates new risks—and new ways to manage them.
Cybersecurity Measures for AI Tools in Auditing
AI systems need serious protection. Encryption, firewalls, secure protocols—they all matter. Regular security assessments catch vulnerabilities before they become problems. Security stays current through consistent updates.
Training makes a big difference. Staff need to spot phishing attempts, understand malware risks, handle data properly. When incidents happen, response plans kick in immediately. Multi-factor authentication adds another layer of protection against unauthorized access.
Ensuring Transparency and Explainability of AI Systems
From a user perspective, it’s important to understand how AI makes decisions. Documentation shows exactly how algorithms work. Regular audits verify that systems follow ethical standards and produce reliable results.
Every output traces back to its input data. Dashboards show results clearly, let users dig into details when needed. The whole system stays open to scrutiny—no black boxes allowed.
Accountability in AI-Driven Audit Decisions
Someone always owns the outcome in AI-driven audits. Clear roles define who handles what. Systems document why decisions got made, what data supported them.
Review processes keep everyone accountable. Regular evaluations show how AI tools perform over time. Communication flows between data scientists, auditors, and executives. Nobody works in isolation.
Emerging Trends and Future Outlook
Audit technology is going to stay on the evolution fast track. Blockchain, IoT, generative AI—they all continue bringing new capabilities to audit. These tools don't just enhance current practices. They fundamentally change what's possible.
The Impact of Blockchain and IoT Technologies
Blockchain changes everything about data integrity and security. Transaction tracing becomes simpler, more reliable. Permanent records reduce errors and fraud naturally. Documentation requirements drop because verification happens automatically.
IoT devices feed real-time data straight into audit systems. Operations monitoring never stops. Teams make faster, better-informed decisions because they see what's happening right now, not what happened last month.
Generative AI's Role in Innovative Auditing
Standard audit tools miss things. They have to—they're limited by programming. Generative AI works differently. It finds patterns hidden in massive datasets, spots problems traditional methods overlook. Auditors spend more time analyzing exceptions, less time looking for them.
Risk assessment improves dramatically. Problems get predicted before they appear. The technology reshapes what audit teams need to know—data analysis skills matter more than ever. Traditional audit skills evolve into something new.
Future Technologies Shaping the Audit Profession
New technologies keep appearing. Firms need to stay flexible, keep adapting. Success depends more on collaboration between auditors and technology experts than ever before. Everyone needs to understand both sides now.
Training never stops. Teams need new skills constantly. The profession keeps evolving, but quality standards stay high. Maybe even get higher. These tools don't just make audits faster—they make them better.
Want to take advantage of your revamped, reinvigorated financial close? Simplify your reporting process? Or streamline your audit preparation? InScope helps finance teams automate manual work, reduce errors, and keep both regulators and stakeholders happy. When you're ready to spend less time wrestling with spreadsheets and more time analyzing results, check out what InScope can do and request a demo today.
FAQs
1. How can AI be used to enhance the auditing process?
AI handles the grunt work in auditing. It processes massive datasets, finds anomalies, flags issues that need attention. This frees up auditors to focus on what matters—analyzing problems, making decisions, thinking strategically.
2. What are some examples of AI technologies currently being used in auditing?
Most audit firms already use AI every day. Machine learning spots patterns in transactions. Natural language processing reads contracts and reports. Automated systems handle basic tasks that used to eat up hours of time.
3. What are the benefits and limitations of integrating AI into auditing practices?
AI makes audits faster, more accurate, better at catching problems early. But it's not magic. The systems need clean data to work properly. They need monitoring to prevent bias. They need humans to interpret results intelligently.
4. How prevalent is the use of artificial intelligence among major audit firms?
Most major firms switched to AI years ago. They use it for everything from basic data analysis to complex risk assessments. Smaller firms are catching up fast—they have to.
5. What impacts might AI have on the future of the auditing profession?
Basic audit work is already automated. That trend continues. Auditors focus more on analysis, less on data gathering. They need different skills now—understanding technology matters as much as understanding accounting.
6. How do AI audit tools differ from traditional auditing software?
Old software followed rules. AI learns from experience. Traditional tools processed what they were told. AI finds problems nobody thought to look for. The difference shows in the results—deeper analysis, fewer missed issues, better insights.